Nephrology Practice Analytics
In recent years, the healthcare industry has undergone significant digital transformation, with the increased implementation of electronic health records and healthcare information systems such as HL7. As a result, it is rapidly becoming data-dependent to deliver coordinated care to patients.
Chronic kidney disease (CKD) is a global public health issue, with more than 37 million people (1 in 7 or 15% of adults estimated) to have CKD in the United States. To better serve CKD patient needs and reduce overall healthcare costs, Panoramic Health has implemented nephrology practice analytics. Big data and AI can be leveraged to drive medical innovation, improve healthcare quality, and create novel ways to cover the unmet needs in nephrology.
Panoramic Health has utilized data and predictive analytics as an essential part of its comprehensive value-based care model to provide nephrologists with a "plug-and-play" model.
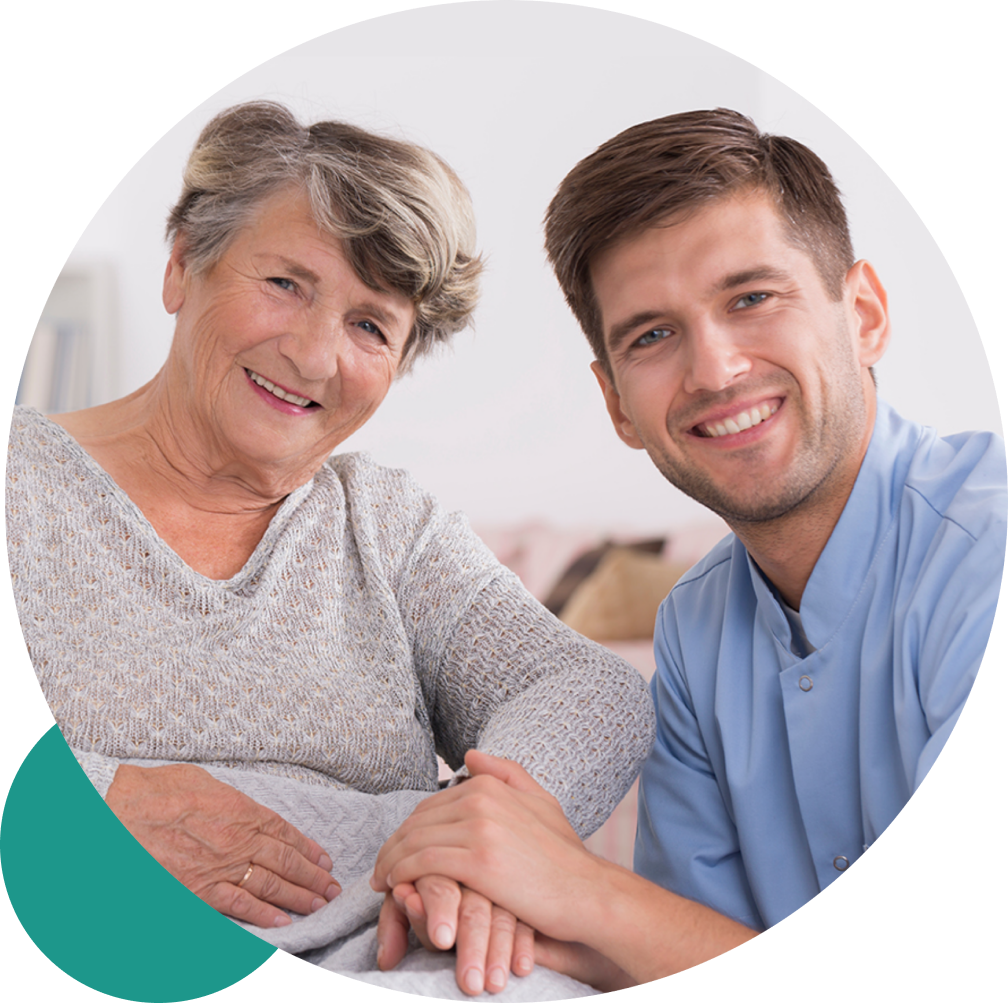
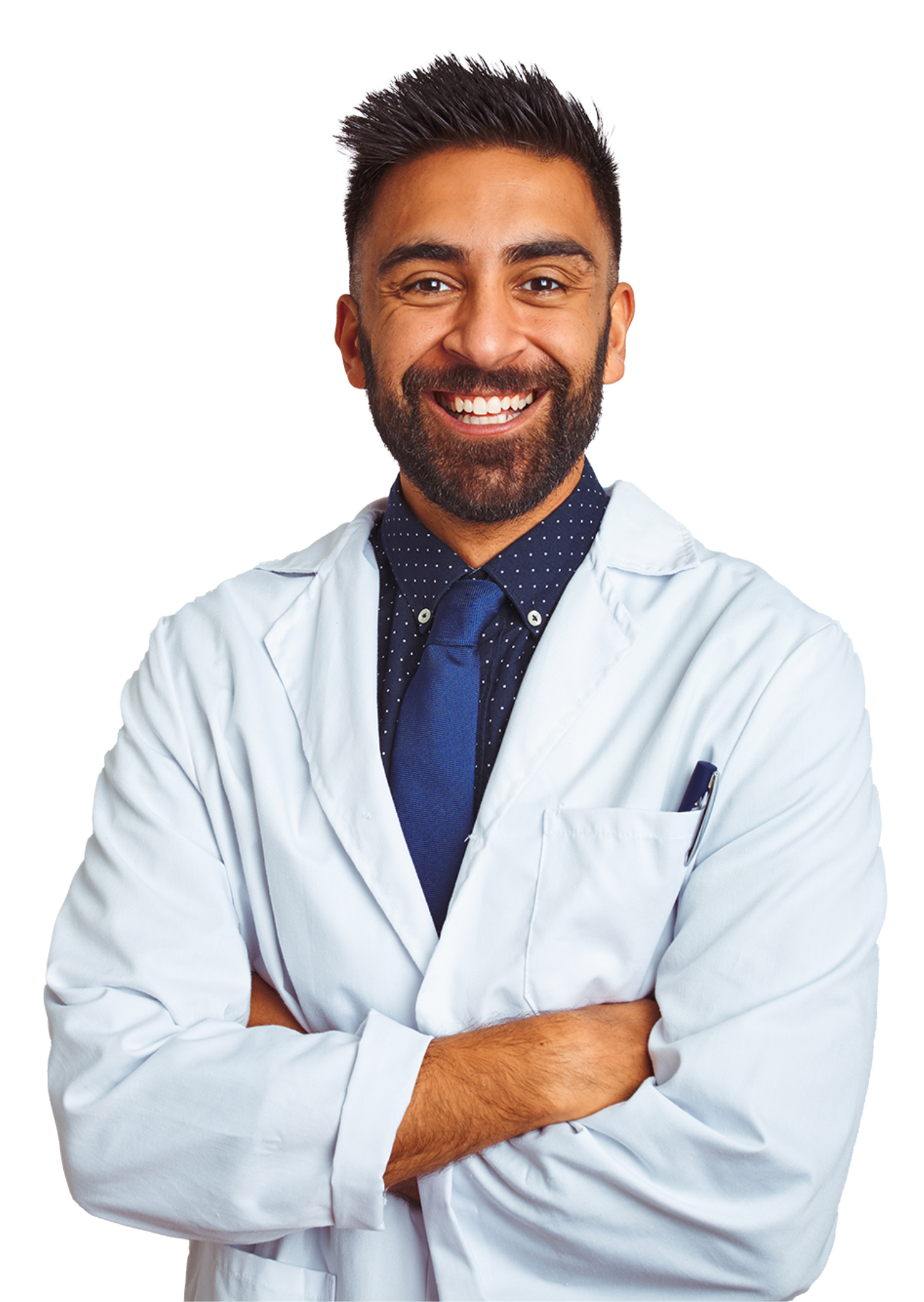
Key Facts:
•Big data in nephrology provides vital data about kidney disease burden, novel risk factors, therapeutic targets, and molecular mechanisms of chronic diseases.
•Sources of big data in nephrology include population surveys, electronic health records (EHRs), patient registries, and open-access clinical trials.
•The curation and standardization of data enable interoperability and facilitate the exchange and consolidation of data from different sources.
•Machine-learning and Artificial Intelligence approaches that use big data can be used for many applications in nephrology, such as early diagnosis and prognosis and clinical decision-support systems.
•Challenges faced in the implementation of and utilization of big data in nephrology relate to the following issues: protection and governance of data, small sample sizes, lack of consistent research funding, and data heterogeneity.
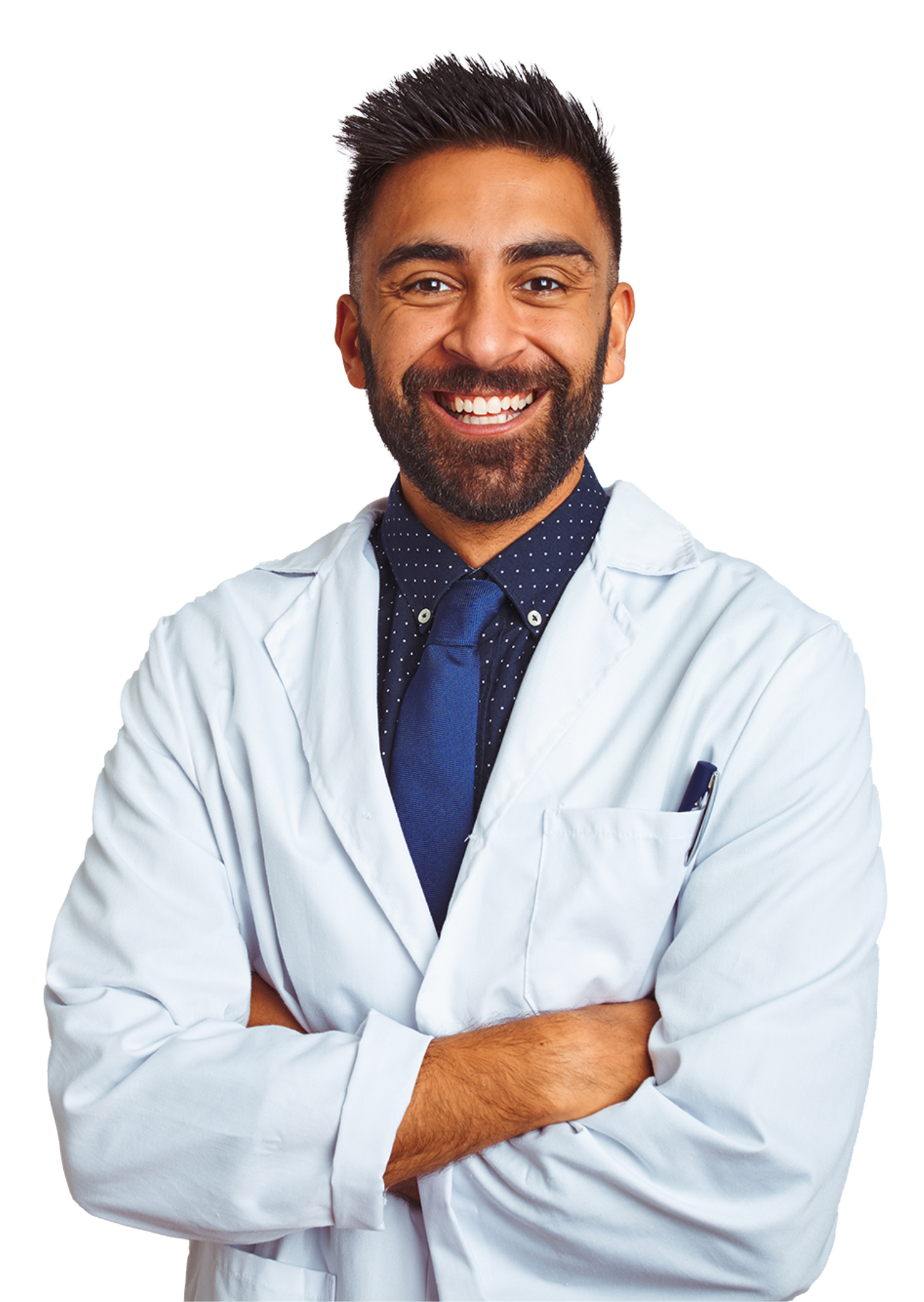
Big Data in Nephrology
Big data can be characterized as a huge array of data that is so complex and large that it becomes problematic to analyze and manage using standard methods and software. In nephrology, there are large amounts of vital data that are continuously collected from patient vitals, laboratory reports, epidemiological studies, clinical trial repositories, mobile health devices and other data from patients with ESRD.
The data collected is often unaligned, temporal in nature and granular (finely detailed). These big data applications can prove challenging to integrate these datasets from different sources in different formats but also provide the opportunity for novel insights into kidney disease to be made.
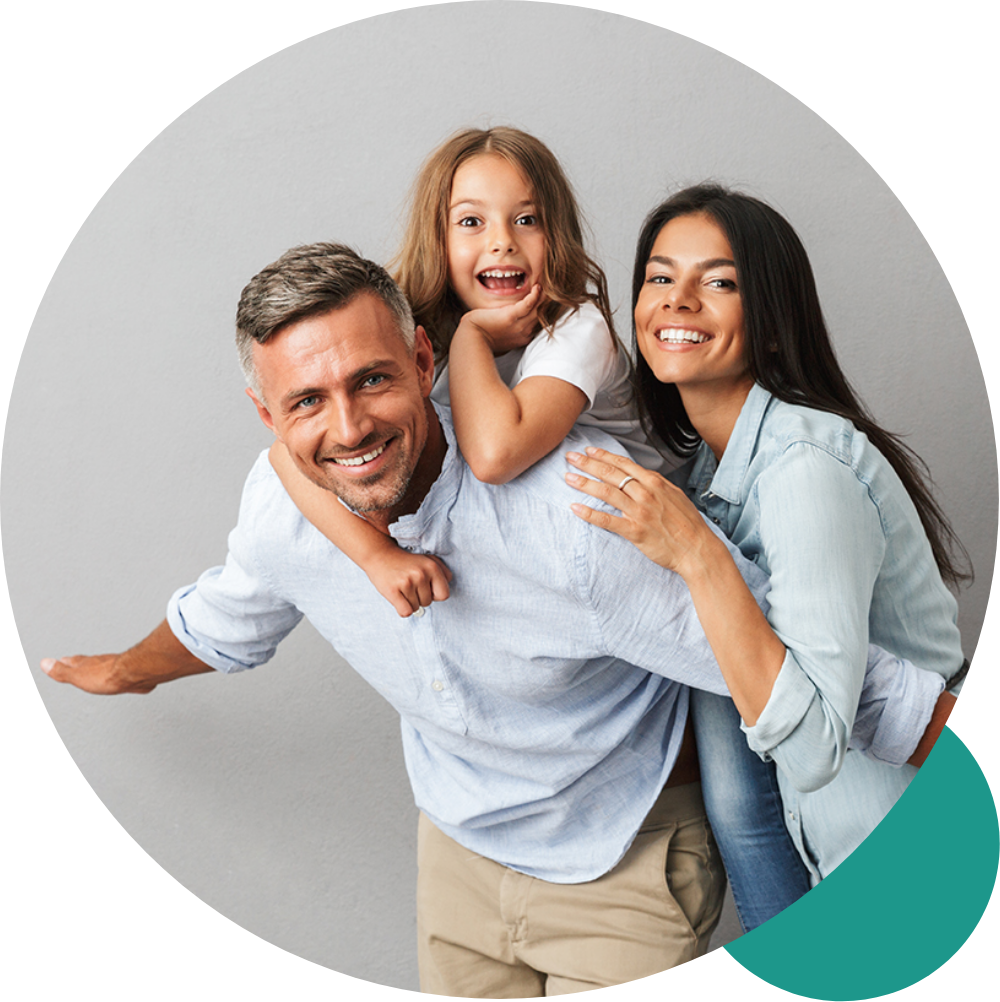
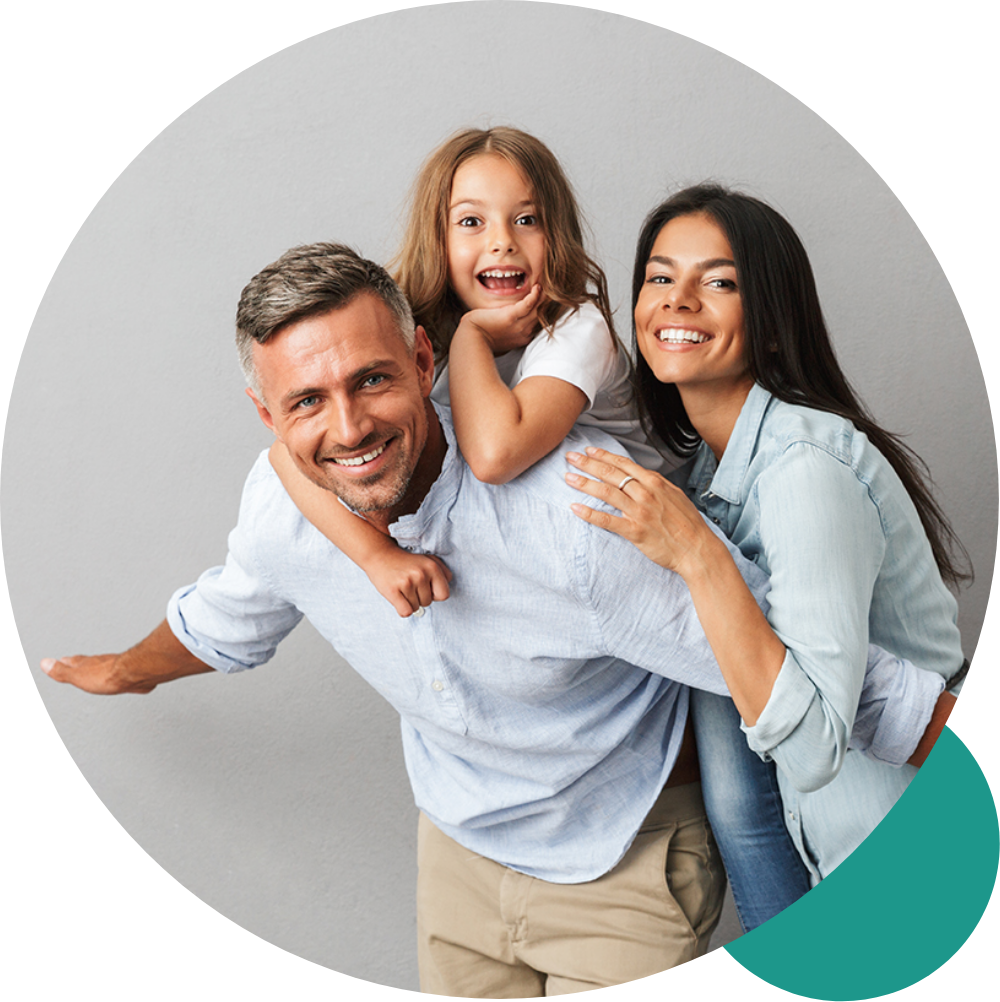
Unmet Needs in Nephrology
Big data has the potential to spearhead medical innovation, improve healthcare quality and reduce overall medical costs for both patients and providers. The unmet needs in nephrology are deep; for example, the global distribution of nephrologists has huge differences in capacity and variation from region to region.
A major challenge to the medical community, including nephrologists, is the large gap between those ready to implement CDSS in their practices and those that are not overly interested in integrating big data into their practices.
Practical Applications of Big Data and AI within Nephrology
The practical applications of big data in nephrology are currently limited compared to other medical Specialties. There is room for significant growth and enhancement of practical analytics applications in nephrology. There are now opportunities for novel insights into kidney disease to be obtained using machine-learning algorithms.
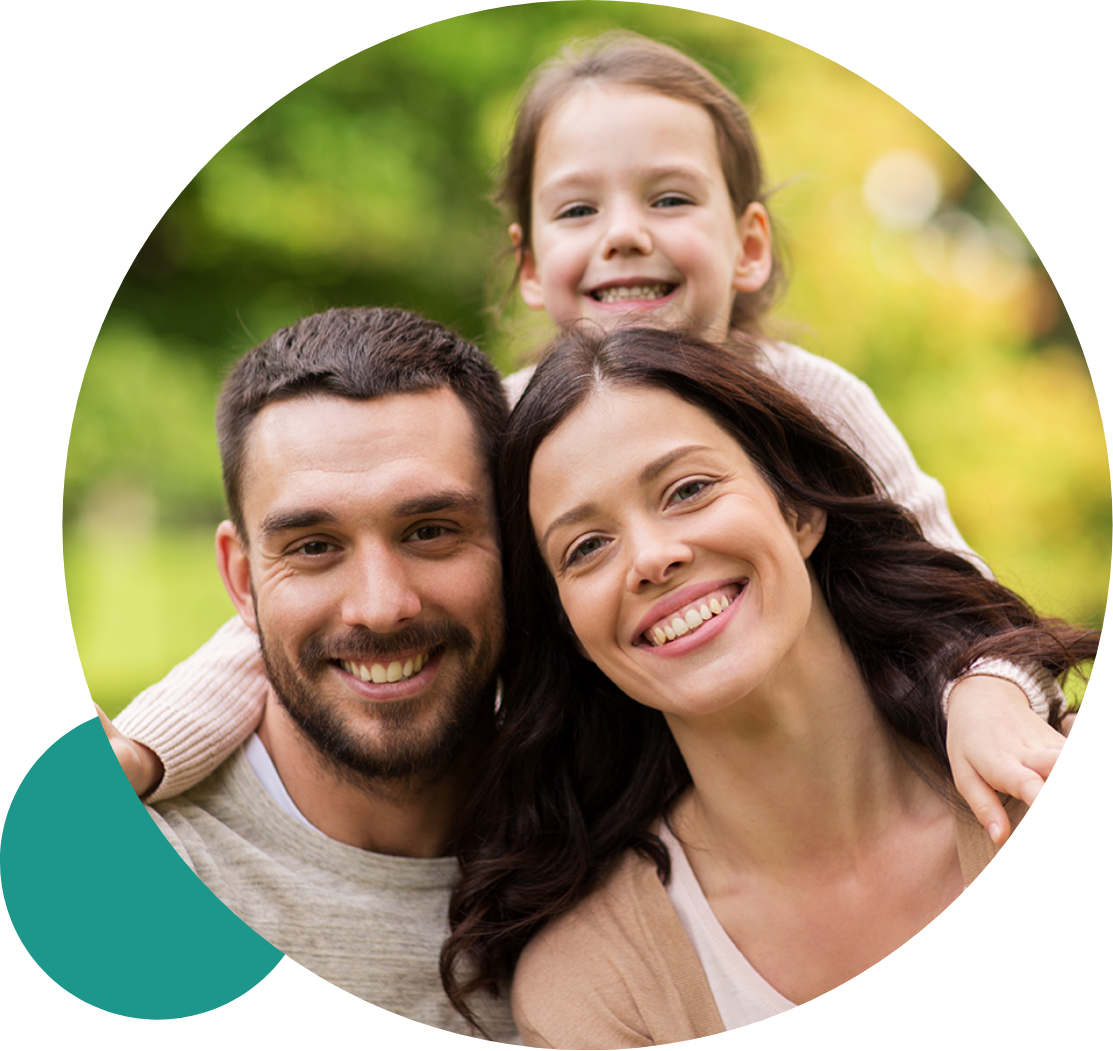
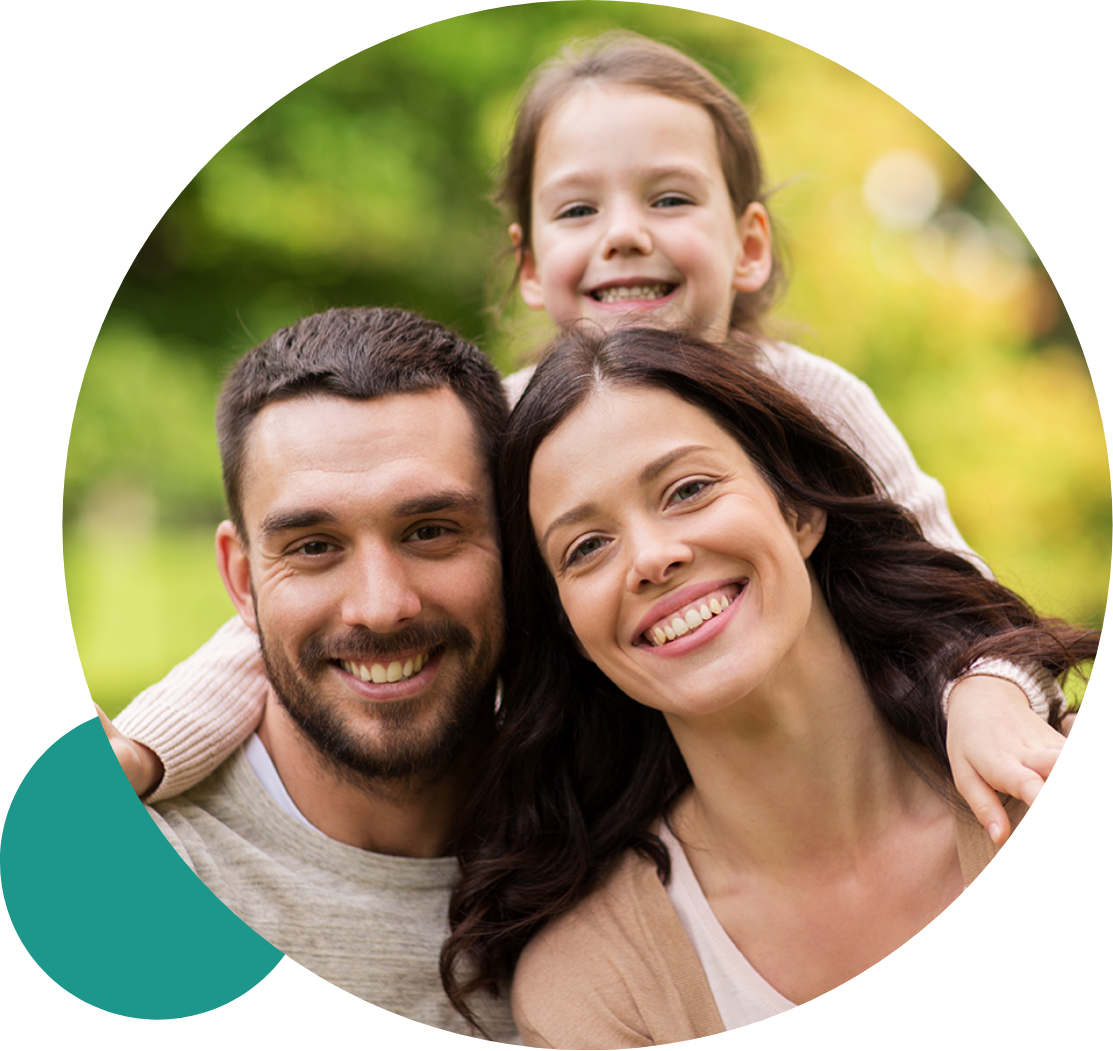
Application of big data to inform decision-making, clinical practice, and innovation
In recent years, thanks to the implementation of machine learning algorithms, risk prediction models have become indispensable for solving many complex clinical problems that arise in CKD and ESRD.
Clinical Decision Support Systems (CDSS) have an important role in the delivery of evidence-based medicine; their algorithms and models are built on real-world patient data. A recent study showed that physician adherence to guidelines regarding the monitoring of CKD could be improved with automated laboratory-based CDSS.
Panoramic Health's ESRD risk predictor can capture more at-risk patients.
Current applications of big data in decision making, innovation, and clinical practice:
•The management of acute kidney injury: classification, detection, and automated alerting of acute kidney injury.
•Clinical decision and risk prediction support: using natural language processing and machine learning for risk prediction of CKD and end-stage renal disease (ESRD).
Potential directions of big data in decision making, innovation, and clinical practice:
•To prompt the activeness of medical imaging: the analysis of urine-formed elements and of renal biopsy images.
•The strengthening of big data-based CDSS and artificial intelligence (AI): the improvement of usability and interpretability of CDSS, and improved/increased collaboration with specialist data scientists.
In recent years, thanks to the implementation of machine learning algorithms, risk prediction models have become indispensable for solving many complex clinical problems that arise in CKD and ESRD. One such model that has been established is the Tangri model, which is a traditional regression model for the prediction of the progression of kidney failure in CKD patients. The data used by Tangri includes routinely available clinical, demographic, and laboratory.
Clinical Decision Support Systems (CDSS) have an important role in the delivery of evidence-based medicine; their algorithms and models are built on real-world patient data. A recent study showed that physician adherence to guidelines regarding the monitoring of CKD could be improved with automated laboratory-based CDSS.
Panoramic Health's ESKD risk predictor can capture more at-risk patients, outperforming the industry standard Tangri model described above.
Current applications of big data in decision making, innovation, and clinical practice:
•The management of acute kidney injury: classification, detection, and automated alerting of acute kidney injury.
•Clinical decision and risk prediction support: using natural language processing and machine learning for risk prediction of CKD and end-stage renal disease (ESRD).
Potential directions of big data in decision making, innovation, and clinical practice:
•To prompt the activeness of medical imaging: the analysis of urine-formed elements and of renal biopsy images.
•The strengthening of big data-based CDSS and artificial intelligence (AI): the improvement of usability and interpretability of CDSS, and improved/increased collaboration with specialist data scientists.
Application of big data in Nephrology research
Population-based nephrology disease surveillance is one of the most essential applications of big data when acknowledging chronic diseases such as CKD. The USRDS is a surveillance system that collects data for the analysis and distribution of chronic kidney disease (CKD) and end-stage renal disease (ESRD). This allows for the tracking of the economic and epidemiologic factors that are closely related to kidney disease.
Panoramic Health has the largest real-time CKD database, with episodic and longitudinal data from over 650,000 patients.
Current application of big data in nephrology research:
•Population-based nephrology research: China Kidney Disease Network and United States Renal Data System (USRDS).
•Data-driven air pollution associated research: to evaluate the link between CKD progression and PM2.5, PM10.
Potential directions of big data in nephrology research:
•To build and establish a CKD surveillance system and a globally or nationally collaborative network. Surveillance systems are based on existing databases and through the sharing of research and data outputs.
•To build real-world cohorts in a practical and profitable manner.
Current application of big data in nephrology research:
•Population-based nephrology research: China Kidney Disease Network and United States Renal Data System (USRDS).
•Data-driven air pollution associated research: to evaluate the link between CKD progression and PM2.5, PM10.
Potential directions of big data in nephrology research:
•To build and establish a CKD surveillance system and a globally or nationally collaborative network. Surveillance systems are based on existing databases and through the sharing of research and data outputs.
•To build real-world cohorts in a practical and profitable manner.
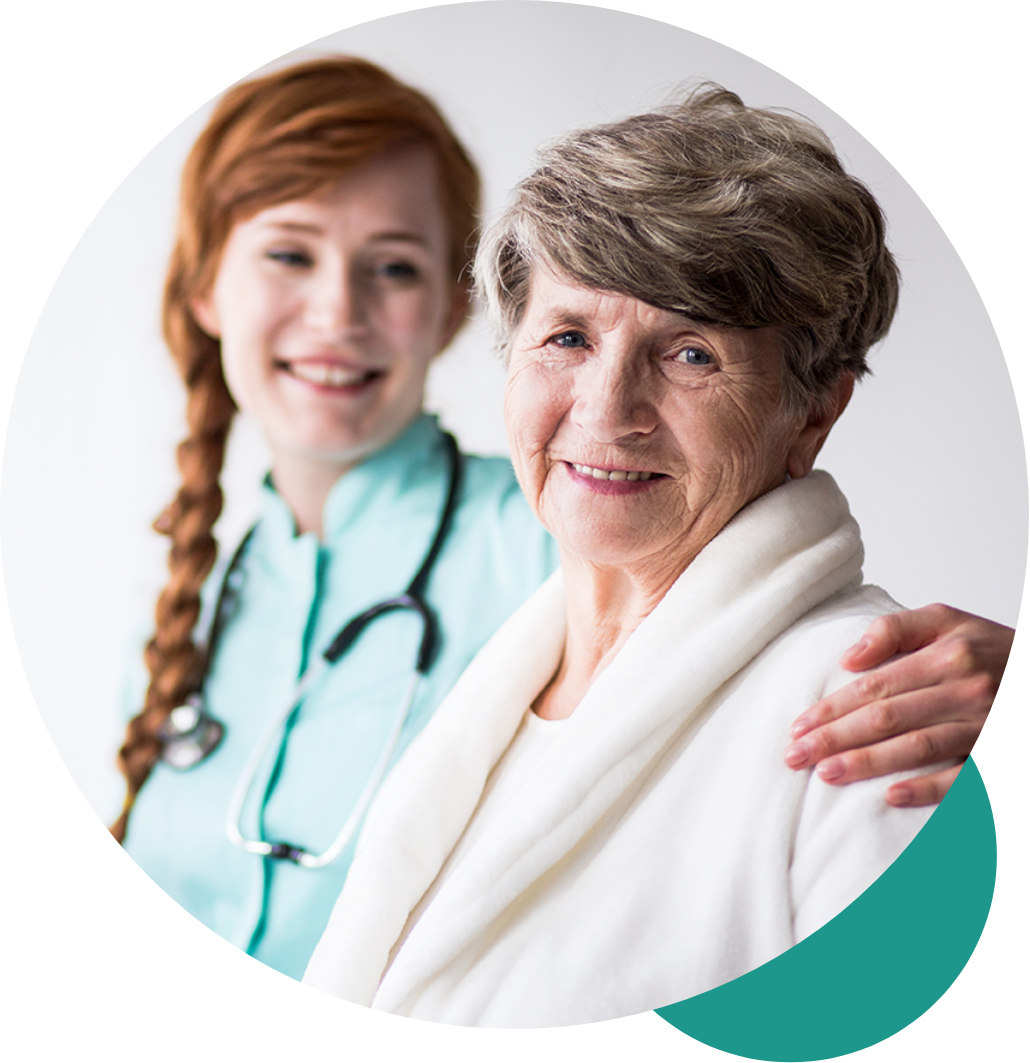
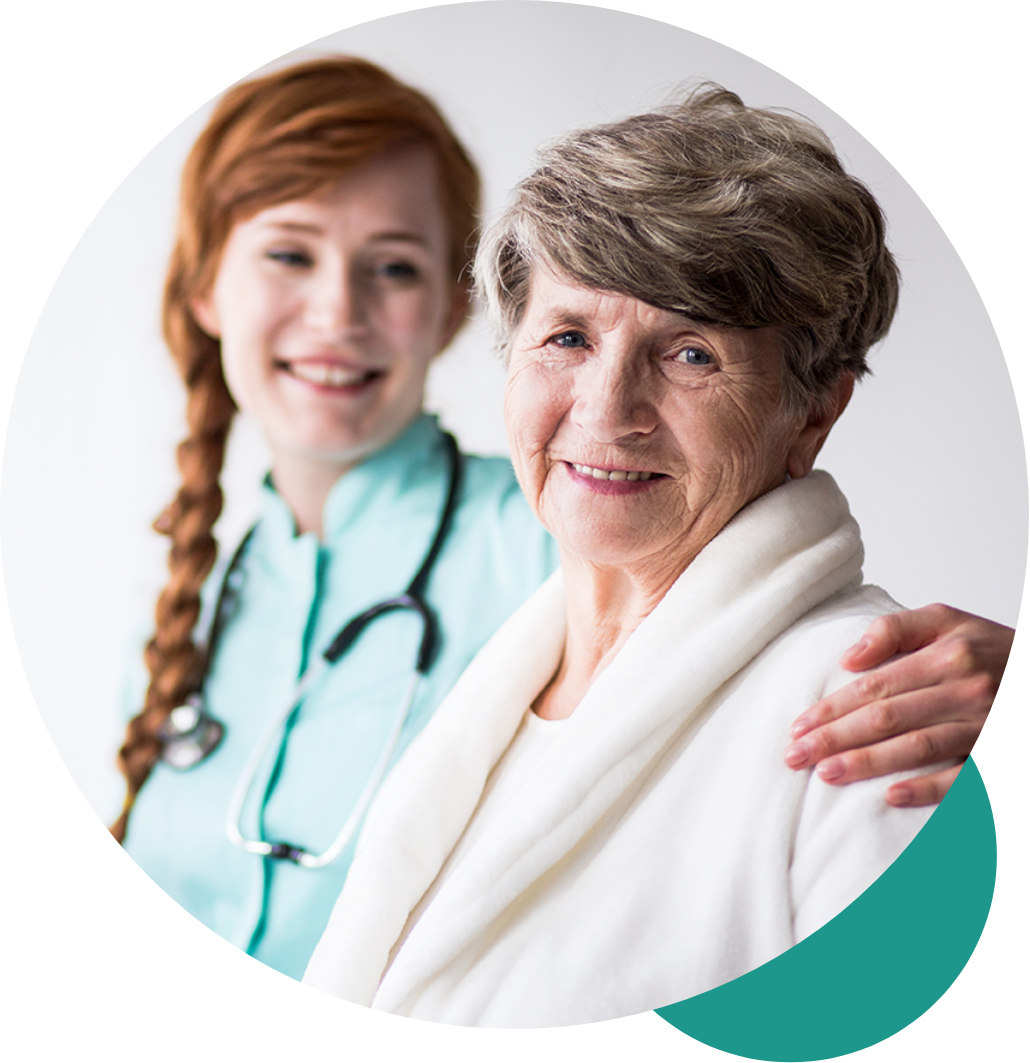
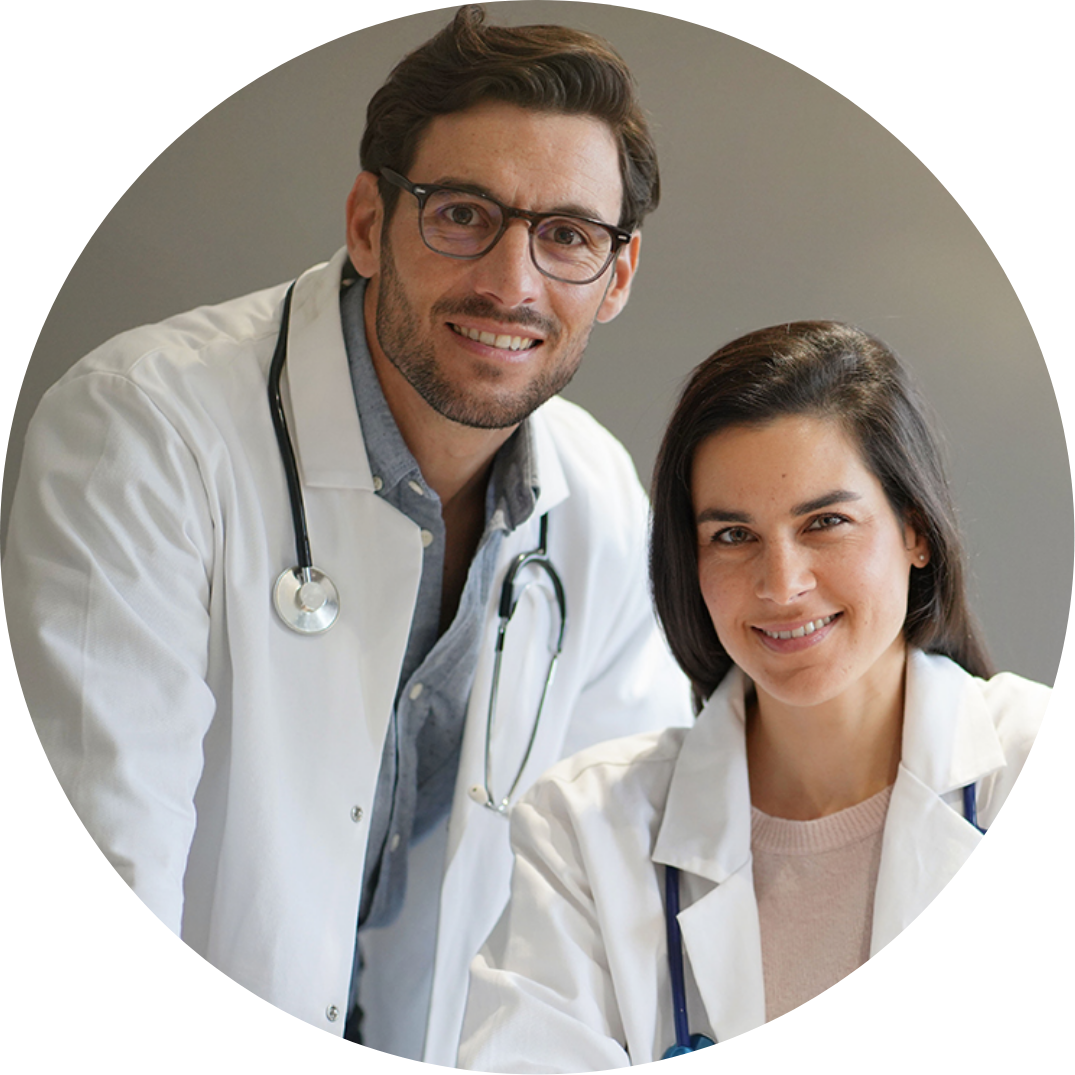
Challenges to overcome
Whilst the integration of practice analytics into nephrology provides many opportunities to improve patient care and research, there are still a lot of challenges that exist when accessing, integrating, and harmonizing data sets in different formats from different sources. Challenges also exist in the protection and security of data, it is essential to ensure patient data is secure and the privacy and rights of the patients and research participants are protected.
When using machine-learning algorithms, it is important to establish that they are able to handle all the small details of kidney health and diseases. Machine learning needs to be able to adapt as medical practices continue to grow and evolve and also be applicable for future and external datasets.
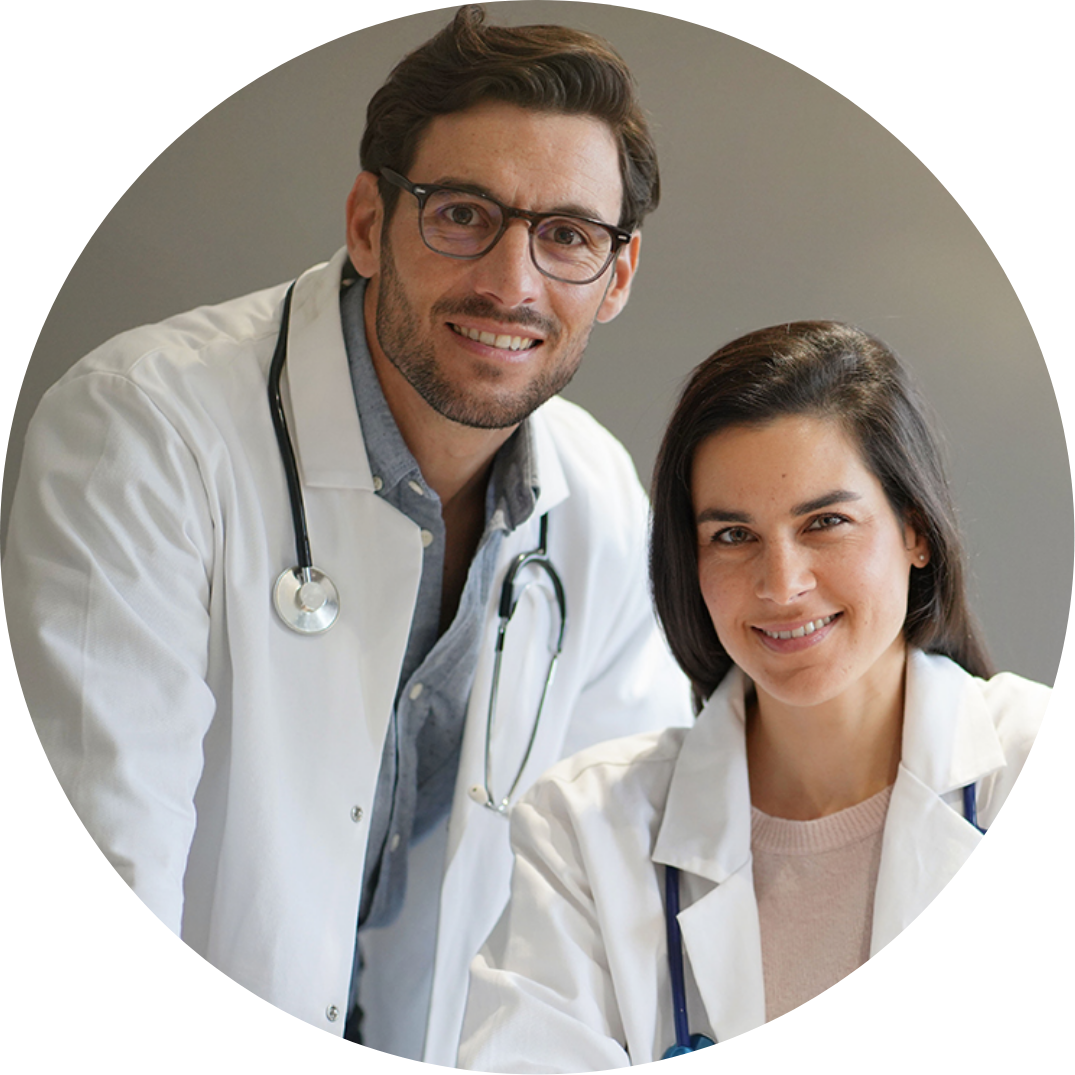
How is Panoramic Health leading the nephrology industry with data and predictive analytics?
Panoramic Health has and makes use of the largest platform of real-time chronic kidney disease databases to power our predictive analytics and data-driven care interventions for patients. This database is comprised of longitudinal and episodic data from over 650,000 patients providing superior analytics and risk stratification. Their dialysis progression predictive model outperforms the industry standard model, capturing more at-risk patients.
Panoramic Health leverages big data to provide patients with customized treatments based on individual risk profiles and CKD stage. This results in the:
•Reduction of hospitalizations
•Understanding patient’s needs
•Potential delay of ESRD
•Accelerated access placement or transplant evaluation
•Reassuring patients and improving their quality of life
•Avoid crash dialysis starts
Our utilization of big data and predictive analytics also provides payers with an enriched understanding of the attributed population through automated reporting. Through our commitment to big data and analytical tools, Panoramic Health is helping CKD patients across the country live longer, healthier lives.
Panoramic Health has and makes use of the largest platform of real-time chronic kidney disease databases to power our predictive analytics and data-driven care interventions for patients. This database is comprised of longitudinal and episodic data from over 650,000 patients providing superior analytics and risk stratification. Their dialysis progression predictive model outperforms the industry standard Tangri model, capturing more at-risk patients.
Panoramic Health leverages big data to provide patients with customized treatments based on individual risk profiles and CKD stage. This results in the:
•Reduction of hospitalizations
•Understanding patient’s needs
•Potential delay of end-stage kidney disease (ESKD)
•Accelerated access placement or transplant evaluation
•Reassuring patients and improving their quality of life
•Avoid crash dialysis starts
Our utilization of big data and predictive analytics also provides payers with an enriched understanding of the attributed population through automated reporting. Through our commitment to big data and analytical tools, Panoramic Health is helping CKD patients across the country live longer, healthier lives.
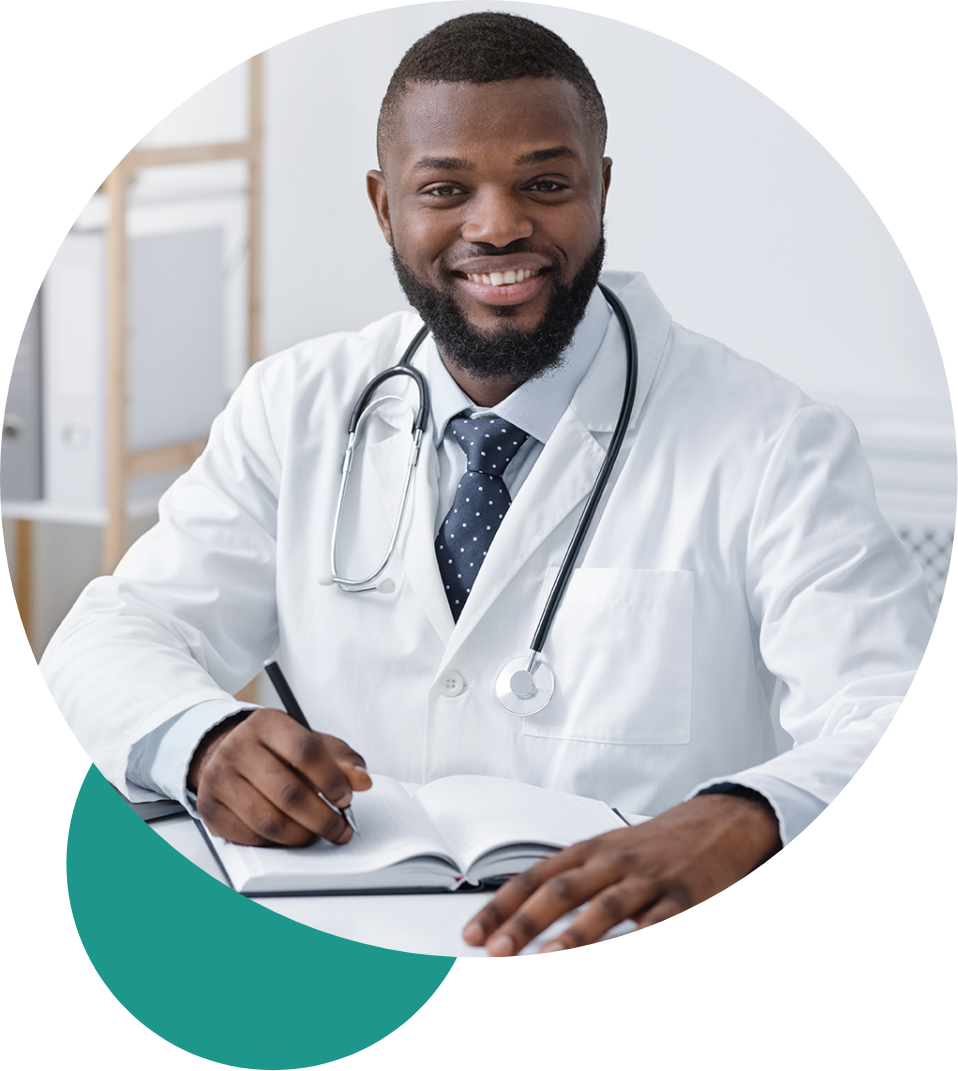
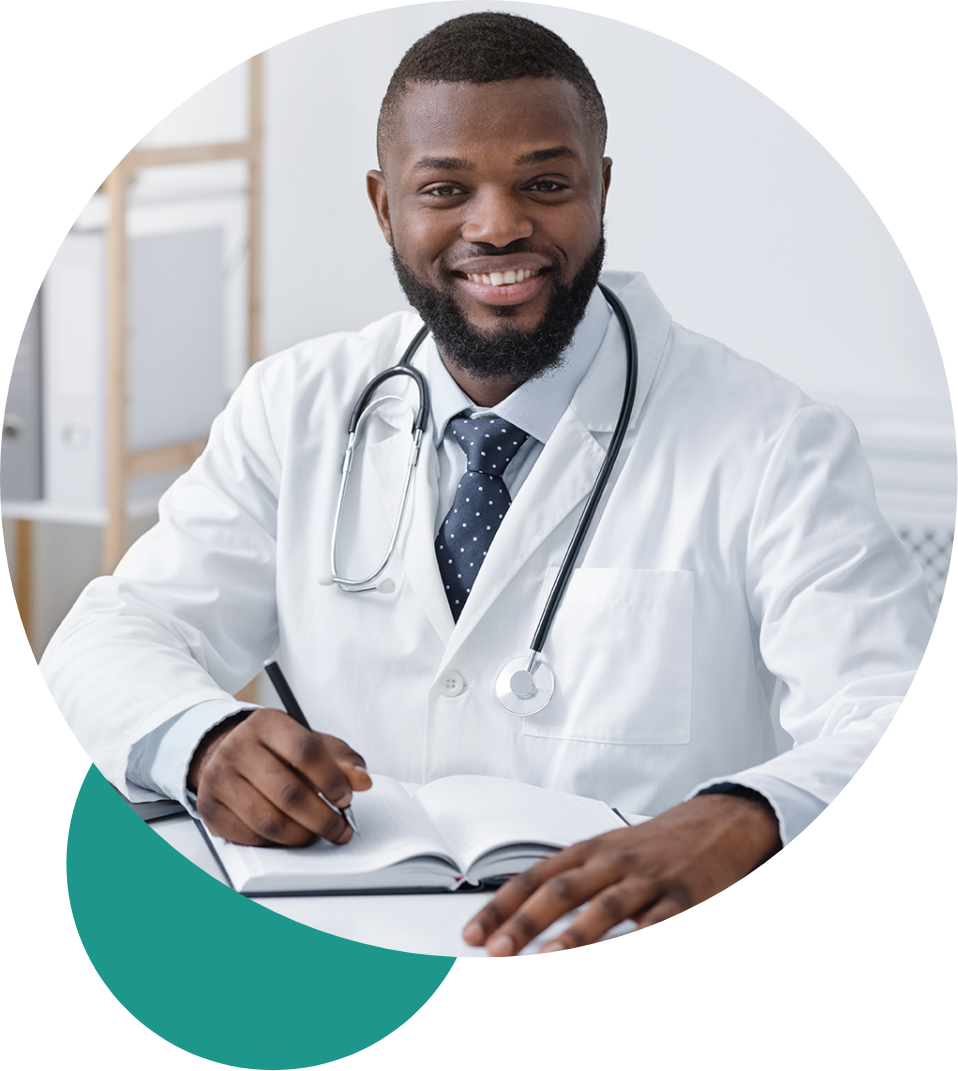